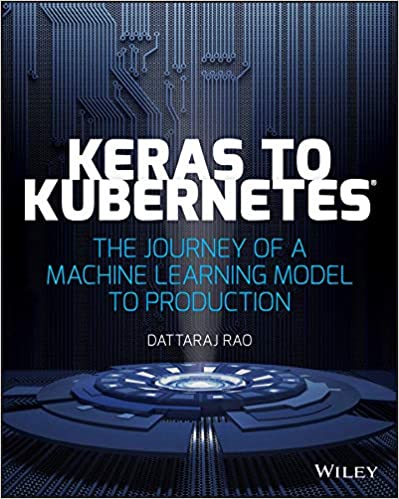
Product details
- Publisher : Wiley; 1st edition (April 16, 2019)
- Publication date : April 16, 2019
- Language : English
- File size : 21203 KB
- Print length : 310 pages
- by Dattaraj Rao (Author)
- Format : epub、pdf
Build a Keras model to scale and deploy on a Kubernetes cluster
We have seen an exponential growth in the use of Artificial Intelligence (AI) over last few years. AI is becoming the new electricity and is touching every industry from retail to manufacturing to healthcare to entertainment. Within AI, we’re seeing a particular growth in Machine Learning (ML) and Deep Learning (DL) applications. ML is all about learning relationships from labeled (Supervised) or unlabeled data (Unsupervised). DL has many layers of learning and can extract patterns from unstructured data like images, video, audio, etc.
Keras to Kubernetes: The Journey of a Machine Learning Model to Production takes you through real-world examples of building DL models in Keras for recognizing product logos in images and extracting sentiment from text. You will then take that trained model and package it as a web application container before learning how to deploy this model at scale on a Kubernetes cluster. You will understand the different practical steps involved in real-world ML implementations which go beyond the algorithms.
• Find hands-on learning examples
• Learn to uses Keras and Kubernetes to deploy Machine Learning models
• Discover new ways to collect and manage your image and text data with Machine Learning
• Reuse examples as-is to deploy your models
• Understand the ML model development lifecycle and deployment to production
If you’re ready to learn about one of the most popular DL frameworks and build production applications with it, you’ve come to the right place!
Editorial Reviews
From the Inside Flap
LEARN HOW TO BUILD A KERAS MODEL TO SCALE AND DEPLOY ON A KUBERNETES CLUSTER
Artificial Intelligence (AI) has, in one form or another, been in existence for over six decades. However, recent years have seen an enormous increase in the amount of collectable data and major advancements in algorithms and computer hardware. Within the realm of AI technology, Machine Learning (ML) and Deep Learning (DL) applications in particular have undergone significant growth. Keras, one of the most popular DL frameworks, can quickly describe a DL model, begin training it on data, and generate more data by modifying existing data. Kubernetes is an application engine that manages applications packaged as Containers, handling all the infrastructure constraints such as scaling, fail-over, and load balancing. With the power, flexibility, and virtually limitless applications of Keras and Kubernetes comes a caveat—they can be challenging to develop and deploy effectively without proper guidance.
Keras to Kubernetes: The Journey Of A Machine Learning Model To Production offers step-by-step instructions on how to build a Keras model to scale and deploy on a Kubernetes cluster. This timely and accessible guide takes readers through the entire model-to-production process, covering topics such as model serving, scaling, load balancing, API development, Algorithm-as-a-Service (AaaS), and more. Real-world examples help readers build a Keras model for detecting logos in images, package it as a web application container, and deploy it at scale on a Kubernetes cluster. A much-needed resource for Keras and Kubernetes, this book:
- Offers hands-on examples to use Keras and Kubernetes to deploy Machine Learning
- Presents new ways to collect and manage data
- Includes overviews of various AI learning models
- Enables readers to re-use examples without modification to deploy models
- Provides clear and easy-to-follow directions
Written by a respected leader in Artificial Intelligence engineering, Keras to Kubernetes: The Journey Of A Machine Learning Model To Production is an ideal book for anyone seeking to learn and apply Machine Learning to their own projects.
From the Back Cover
LEARN HOW TO BUILD A KERAS MODEL TO SCALE AND DEPLOY ON A KUBERNETES CLUSTER
Artificial Intelligence (AI) has, in one form or another, been in existence for over six decades. However, recent years have seen an enormous increase in the amount of collectable data and major advancements in algorithms and computer hardware. Within the realm of AI technology, Machine Learning (ML) and Deep Learning (DL) applications in particular have undergone significant growth. Keras, one of the most popular DL frameworks, can quickly describe a DL model, begin training it on data, and generate more data by modifying existing data. Kubernetes is an application engine that manages applications packaged as Containers, handling all the infrastructure constraints such as scaling, fail-over, and load balancing. With the power, flexibility, and virtually limitless applications of Keras and Kubernetes comes a caveat―they can be challenging to develop and deploy effectively without proper guidance.
Keras to Kubernetes: The Journey Of A Machine Learning Model To Production offers step-by-step instructions on how to build a Keras model to scale and deploy on a Kubernetes cluster. This timely and accessible guide takes readers through the entire model-to-production process, covering topics such as model serving, scaling, load balancing, API development, Algorithm-as-a-Service (AaaS), and more. Real-world examples help readers build a Keras model for detecting logos in images, package it as a web application container, and deploy it at scale on a Kubernetes cluster. A much-needed resource for Keras and Kubernetes, this book:
- Offers hands-on examples to use Keras and Kubernetes to deploy Machine Learning
- Presents new ways to collect and manage data
- Includes overviews of various AI learning models
- Enables readers to re-use examples without modification to deploy models
- Provides clear and easy-to-follow directions
Written by a respected leader in Artificial Intelligence engineering, Keras to Kubernetes: The Journey Of A Machine Learning Model To Production is an ideal book for anyone seeking to learn and apply Machine Learning to their own projects.
About the Author
DATTARAJ JAGDISH RAO is a Principal Architect at GE Transportation (now a part of Wabtec Corporation). He has been with GE for 19 years working for Global Research, Energy and Transportation. Currently, he leads the Artificial Intelligence (AI) strategy for the global business, which involves identifying AI-growth opportunities to drive outcomes like Predictive Maintenance, Machine Vision and Digital Twins. He is building a Kubernetes based platform that aims at bridging the gap between data science and production software. He led the Innovation team out of Bangalore that incubated video Track-inspection from idea into a commercial Product. Dattaraj has 11 patents in Machine Learning and Computer Vision.
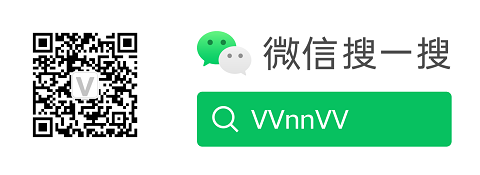